How Commercial Property Identification Can Fuel Underwriting Innovation
This is the second post in our series, Bringing Innovation to Commercial Property Lines. Missed the previous posts? Read Part I here and Part III here.
In our last blog post, we dove into the complexities and breadth of commercial property risks, why innovation in the sector has been slow thus far, and why we think this is the decade where all that will change. We also touched on one of the chief obstacles to digitization in commercial property insurance: the standardization and accuracy of basic property data.
“…for most commercial lines carriers, fundamental obstacles remain before widespread adoption and balance sheet gains can be realized. For one, inconsistencies in basic property facts make it challenging to build advanced or automated decisioning frameworks for better risk selection.”
Property insurance data starts with an address. Given unlimited time and resources, it’s easy to visit a property, walk around, and compare a location and its structures with the information provided in a building schedule. But even then, errors occur—portions of a property may not be visible or accessible, or an inspector may include incorrect structures. As we built our commercial lines solution, we spent hundreds of hours speaking to underwriters and heard many stories of carriers receiving a claim for a building they didn’t know was covered, leading to premium leakage. Others had included wrong buildings as part of a property, incorrectly assessing the overall risk.
Building identification is the foundational element of property underwriting. If an underwriter is not assessing the right building, all the subsequent data and geo-enrichment are irrelevant.
Today, many insurers are attempting to assess properties remotely using aerial imagery, ostensibly to increase decision speed and operational efficiency. However, manual aerial imagery reviews present their own challenges. For example, an underwriter may paste an address into Google Maps then wonder whether the pin dropped on the right building, where the property actually starts and ends, which structures are part of the property under evaluation, and when that aerial picture was taken. A commercial property parcel may be oddly shaped, structures may be connected and tough to disambiguate, or one structure may have multiple businesses. Imagery alone cannot answer these questions and underwriters must spend a great deal of time matching buildings in imagery to various data fields in an application.
For highly accurate, automated property identification, additional data fusion is necessary. In creating a powerful commercial property solution, Cape Analytics has developed a process for getting underwriters to the correct property every time. We do this through an advanced matching logic engine that fuses geocoding, aerial imagery analytics, and additional third-party information to understand the truth on the ground at specific points in time, which can then be confirmed visually in a browser-based application.
To demonstrate the power of this solution, we’ll walk through a few challenging property examples submitted by existing customers and then describe how Cape’s engine solves each issue.
Building identification for large arbitrary areas
Underwriters for large habitational properties often spend up to 30 minutes in Google Maps attempting to identify all the structures from a building schedule submitted by the agent. The example below shows why this is so time-consuming and error-prone. This HOA has buildings on 11 different streets with different shapes and sizes. It’s not obvious which of the 75 buildings listed in the statement of value belong to the HOA.
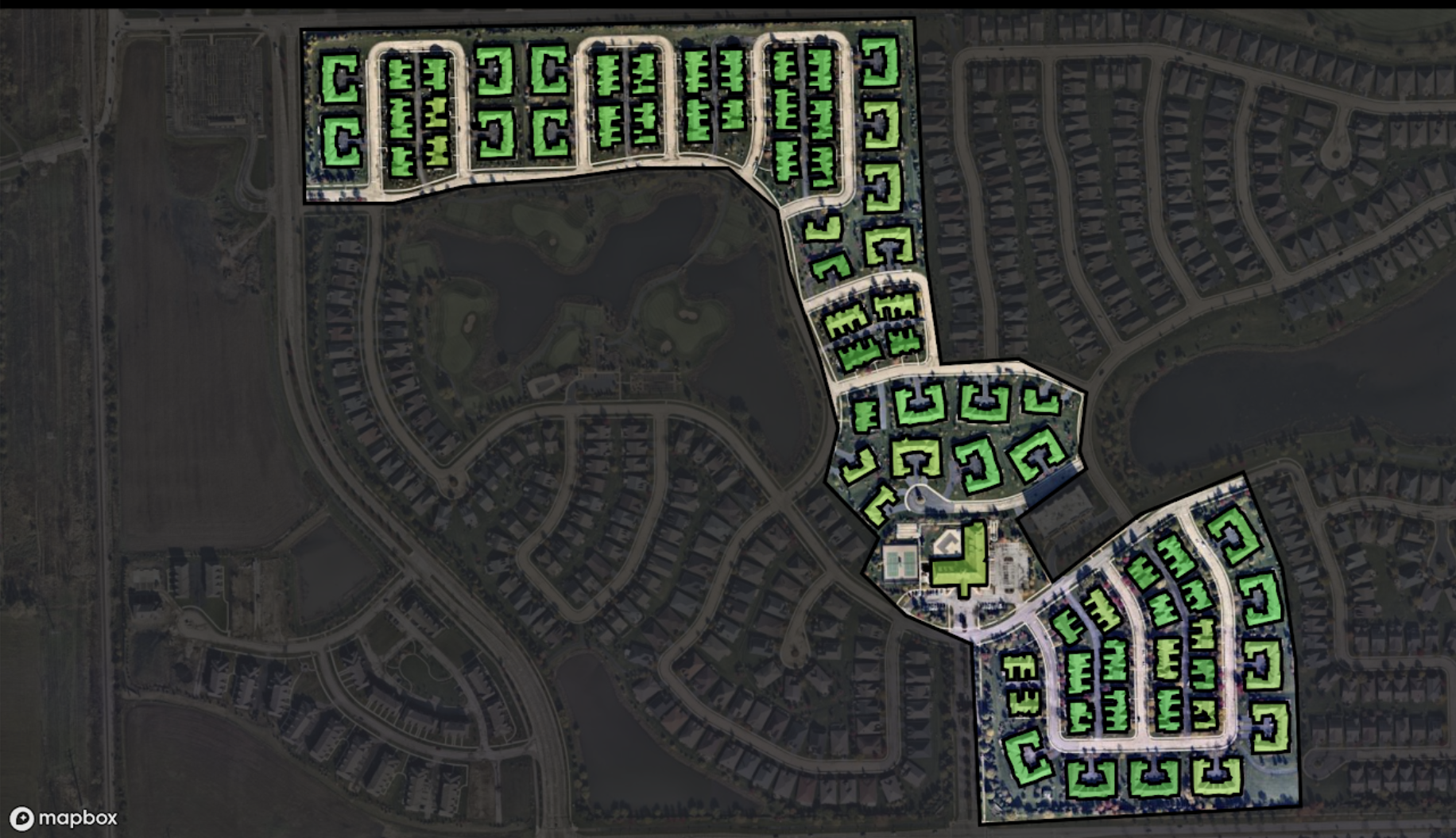
To solve this issue, we invested heavily in building an incredibly robust property-matching solution. We leverage multiple location data inputs—address, building footprints, and other third-party information—and combine them in an optimal manner for insurance risk assessment. In doing so, we’ve improved the accuracy of our building identification results to a near-perfect 98 percent.
For this kind of property, Cape’s browser-based application can reduce the time it takes to correctly match buildings to a risk by more than 90 percent. For the example property above, an underwriter may complete this step in less than two minutes, saving 25 minutes on a single submission. An underwriter simply copies the addresses from a building schedule into the application, then confirms or modifies the pre-populated property boundary created by Cape’s automatic property-matching engine. For the truly challenging corner cases where the underwriter may disagree with the automated boundary, the application allows users to alter boundaries and feed the correction back into the Cape system.
Identifying an attached building segment that belongs to a business
Underwriters using digital tools often have a hard time resolving and attributing different structures when they may share a wall with a property on an adjacent structure. While the task becomes easier upon review of top-down imagery, it’s often not immediately evident which structure (or roof segment, as seen from overhead) corresponds to which property.
Cape has developed a deep learning model that considers visual queues in overhead aerial imagery, supplemented with spatial information corresponding with property ownership, to consistently and accurately define the extent of a given property’s structure(s) and attribute it to a given risk. The example below would be difficult to resolve from the ground or with overhead imagery alone, but Cape’s model accurately assigns several visually different roof segments to a single property. This model performs with higher than 90 percent accuracy, exceeding human performance at the same task.
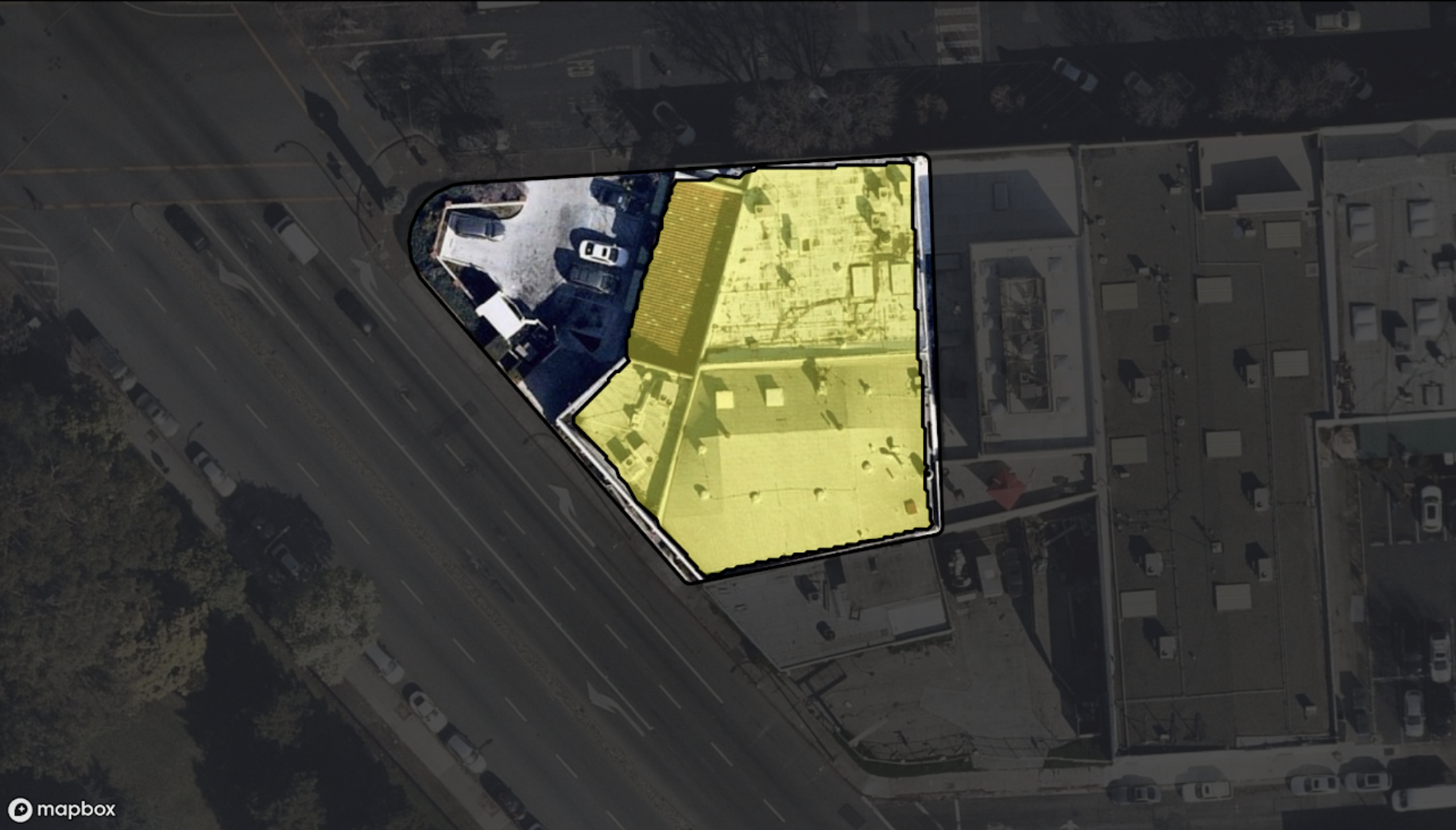
Identifying all the buildings that belong to a property
The final challenging case our customers identified is one in which several non-connected buildings all belong to a single insured property. This is often seen in commercial areas where a business has multiple structures on the same or adjacent properties. Many underwriters find it challenging to digitally identify which buildings actually belong to the business, especially when they have dissimilar footprints and construction types.
The example below is a logistics business with a large warehouse, a customer center, and an administrative office. Neighboring businesses also have similar structures, making it easy to erroneously include an extra building, producing a greatly overestimated insured value—and likely lost business due to an over-inflated, uncompetitive premium. Again, in this case, Cape fuses multiple data sources to identify all the structures that belong to the business, making it as easy as entering an address into the Cape application to arrive at the spatial property delineation you see in the example below. Further, review in the app enables the underwriter to consider the entire spatial extent of the property as they look for additional sources of risk.
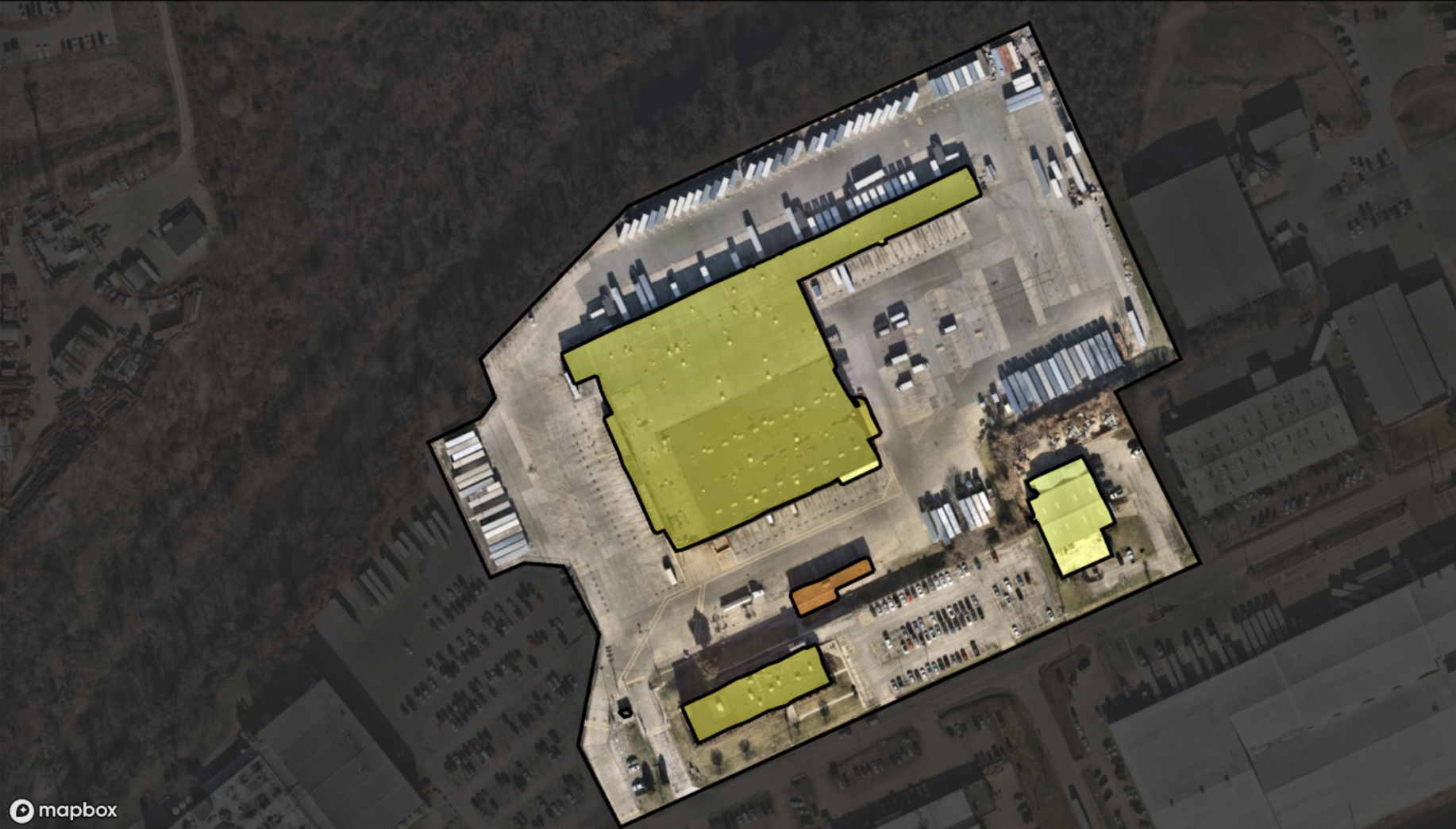
Creating a new, reliable data foundation
Getting property definitions and building identification right is critical so the underwriter may truly understand each risk, without inadvertently exposing the carrier to premium leakage or overcharging. Future blog posts will talk about the value of our automated property condition solution for improved rating—but enabling the underwriter to quickly and reliably identify buildings is step one. With high confidence in matching buildings and property extents to the risk being evaluated, underwriters can then consider additional loss-predictive information—the condition of the roof, the parking lot, storage areas, etc —to more effectively direct additional onsite inspectors and more appropriately price the policy. Getting the location right is a first-order problem—doing so in an automated and reliable way is the foundation that allows carriers to automate underwriting.
At Cape, we focus on designing products and solutions in a way that allows carriers to automate certain aspects of an underwriting workflow or even the process in its entirety. The same is true for building identification. We used a lot of visuals in this blog post to show examples of the problems we solve, but it’s important to note that all the underlying data is available through APIs, batch processing, as well as within our commercial underwriting app. Cape’s commercial lines product offering adapts to wherever a carrier is in on the automation journey and we pride ourselves on the deep partnerships we form with our customers that will help them navigate the next decade of innovation.