The Underinsurance Problem: Root Causes and an AI Solution for a Way Forward
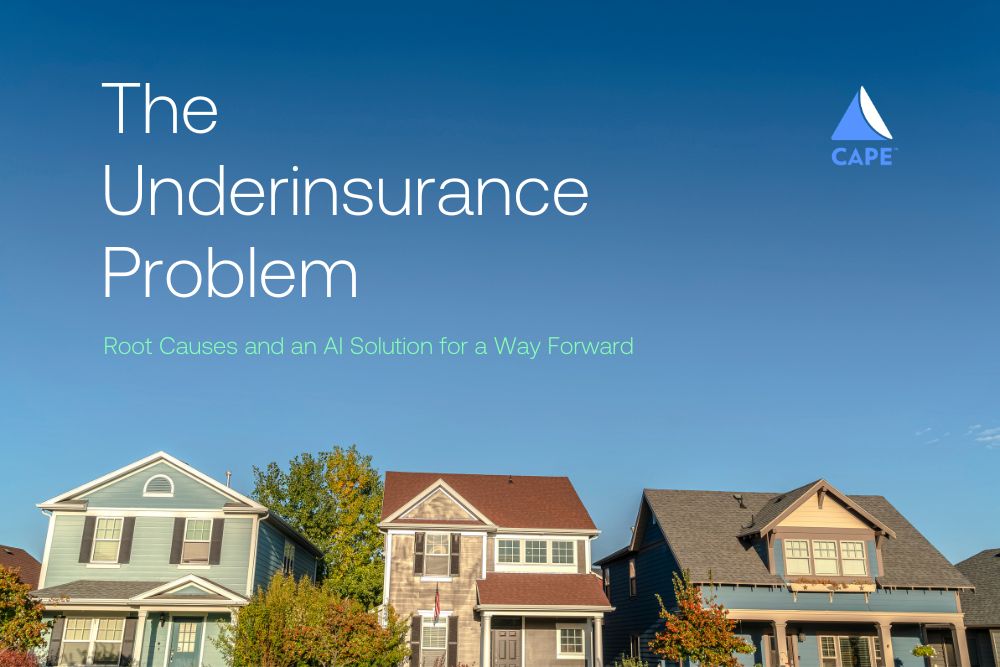
The fact is as simple as it is perplexing: if someone owns a home in the U.S., odds are that their property is underinsured.
In a staggering statistic, approximately 2 out of every 3 homes in the U.S. are underinsured. On average these homes are underinsured by 22%, but some homes can see underinsurance amounts higher than 60%.
What does it mean to be underinsured? It means that the amount of insurance coverage received is less than the value it would take to rebuild the home. While this might mean that homeowners can enjoy lower monthly premiums, the consequences can be life-altering in the event of a natural disaster or total loss scenario.
Nowhere has this been more apparent than in the wake of several large wildfires over the last decade. Almost 80% of homes that were affected by the 2018 California wildfires were underinsured, with 60% of those homes being severely underinsured. More recently, the surprise Marshall Fire in the closing days of 2021 led to 951 total loss claims where up to 67% of homes were underinsured. The Colorado Division of Insurance estimated that this quick, two-day blaze saw an underinsurance gap of up to $155 million.
In an insurance marketplace that has successfully served its customers for years, how does this happen?
Underinsurance: Root Causes
Underinsurance is caused by both technological and human factors. On the technology side, the coverage software can misinterpret unstandardized and disparate public data sources to produce inaccurate data that provides a shaky foundation for the rest of the process. Software shortcuts and the reliance on only a few basic informational questions to deploy coverage also lay the groundwork for widespread underinsurance. Automated processes may also rely on customer-input information, which can often be inaccurate.
Technology/Data Factors | Human/Process Factors |
– The underlying data is inaccurate – Software misinterprets public data sources – Replacement cost estimation software allows for shortcuts that lead to underestimating – Software requires time and expertise to use effectively, but typical deployments rely on a few questions | – Producers are incentivized to streamline new quoting and renewals by not raising issues that risk losing customers or increasing premiums – Lack of expertise – Replacement cost estimations are not reviewed or updated at renewal and get worse over time – Failure to keep up with inflation – Inaccurate agent inputs |
Human factors are also contributing to the problem, where larger macro issues are at play. Because coverage is often compared solely by the price of the premium, producers are pressured to streamline the new quoting process and not raise issues that might lose customers in a competitive marketplace where an attractive premium is king. This means that in the quest to provide a competitive quote and acquire a customer, accurate coverage amounts become a secondary consideration. This same force is also in play when it comes to renewals, where insurers are pressured to keep premiums, and therefore coverage amounts, low in order to retain a policyholder in an easily-shoppable market. But as consumers become increasingly aware of the underinsurance issue, the carriers that do right by the homeowner and provide sound advice will win out over the carriers that build a reputation for stranding customers in the event of a catastrophic loss.
Tackling Underinsurance with Accurate AI-Backed Data
The issue of underinsurance can be tackled head-on by addressing one of the most significant root causes of the problem: inaccurate foundational data.
CAPE Analytics has found that as many as 25% of properties in a typical carrier’s book contain substantial errors for three highly influential drivers of replacement cost: living area, quality grade, and the number of stories. These are the errors that result in substantial replacement cost estimation and coverage mismatches and can unknowingly impact both carriers’ financials and insureds’ ability to be properly covered.
CAPE’s Primary Property Elements are fundamental AI-verified property data points that carriers can confidently rely upon. Thanks to Foundry, the company’s new data fusion and machine learning platform, CAPE ingests an array of imagery, traditional property, and unconventional data sources to produce accurate and dependable property insights that can drive better replacement cost estimations.
Designed to improve the accuracy of replacement cost estimation inputs, the technology behind Primary Property Elements adds a layer of transparency that can allow carriers to use them in high-confidence scenarios when confidence in the existing data sources is low.
With an assist from AI and machine learning, the industry can begin to directly address the underinsurance problem and help homeowners properly protect their most important investment.
To learn more about CAPE’s Primary Property Elements click here.
Aggregate Statistics Created Using Data Produced from Nearmap Imagery and Other Data Sources