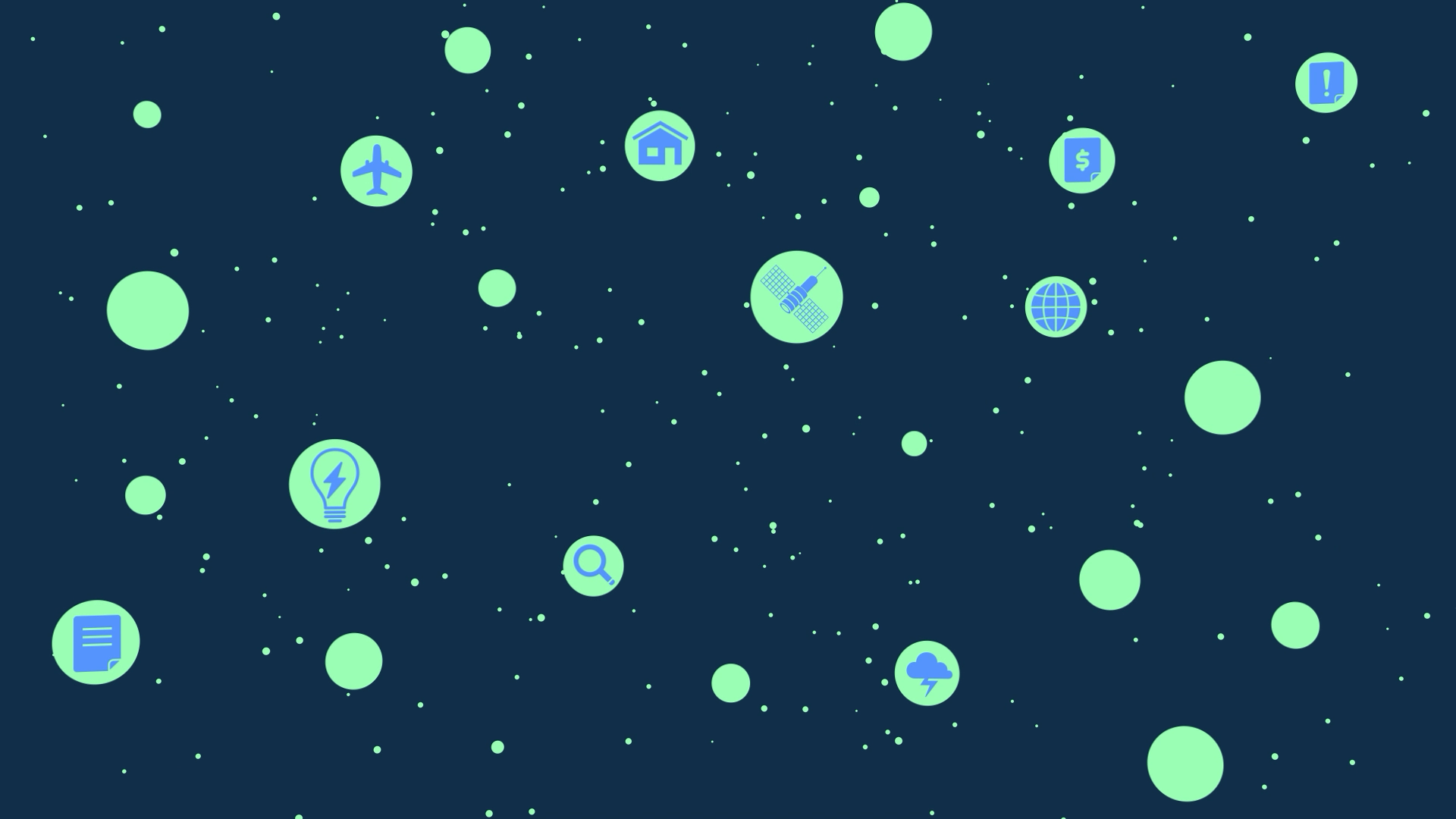
Insurance Property Data: Scaling Mountains & Closing Gaps With AI-Powered, Multisource Data
Insurers relying on standard data providers to expedite the quoting process have long been undermined by incomplete or inaccurate data that can lead to widespread underinsurance or costly mistakes across coverage, pricing, and replacement cost calculations. But today, a new generation of machine learning-based, multisource property data solutions is changing that.
One of the most significant benefits of conventional data sources has always been the savings in time and effort required to fill out applications manually. But that speed and convenience have come at the cost of data accuracy. It’s not unusual for homeowners to be unaware of the type and age of their roof, or details like the home’s distance to a fire station. What’s more, data provided in quoting workflows is very often wrong about these and countless other property details used in replacement cost estimates (RCEs).
These errors result in significant RCE and coverage mismatches that can negatively impact both carriers’ financials and insureds’ ability to be adequately covered. But it doesn’t have to be this way.
The Foundational Data Challenge
Over the last few years, high-quality data on property characteristics, the prospective customer, replacement costs, and more have become widely available through public records, credit bureaus, analytics providers, and other sources. This includes up-to-date property intelligence acquired via high-resolution aerial and satellite imagery.
Yet few carriers interrogate and utilize this data to its full potential, and fewer still can operationalize it effectively on their own. The consequences include continued reliance on inaccurate data that can lead to excessive exposure when quotes are too low and premium loss when they’re unnecessarily high. It also propagates dangerous levels of underinsurance and coverage mismatches.
Take last winter’s Marshall Fire in Colorado, for instance. Four out of five policyholders reporting total property losses were underinsured—to the tune of more than $700 million. No wonder more than 60% of US homes are “woefully underinsured” against wildfire—or any other peril for that matter. Factor in the impact of rampant inflation, and these problems are only compounded.
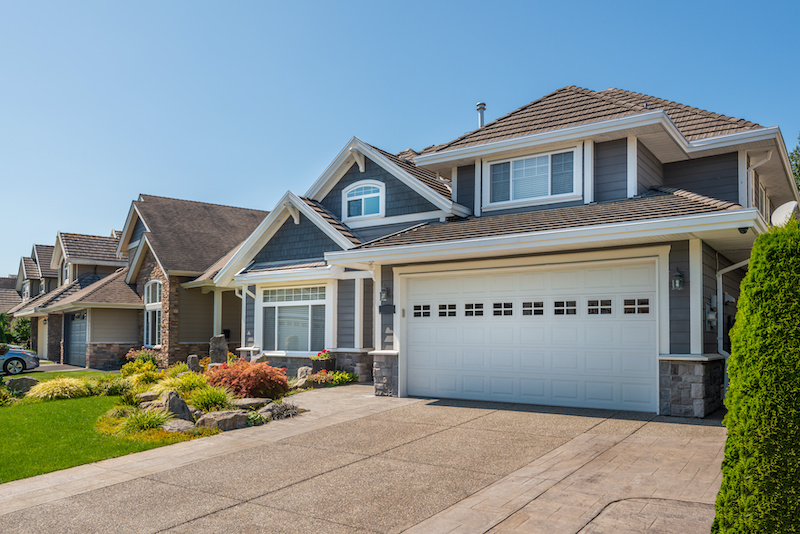
At CAPE, we realized a new approach to this foundational issue was needed. An array of data sources must be brought to bear to match pricing to exposure and close the coverage gap. By layering in information from numerous sources, for instance, it’s possible to recognize commonalities in data pertaining to specific property characteristics that allow you to have a high level of confidence about the accuracy of your property information. As we’ve discovered, having data consistency across sources is beneficial to verifying information—but conflicting data can be high-value, too.
Using machine learning, you can cull through internal and external sources and recognize data that may be less reliable. The accuracy of living area estimations can vary wildly county by county, for instance. You can also determine when inaccurate data has been propagated across multiple sources and rendered them all less reliable. And you can verify each data point from different perspectives, including aerial imagery. This way, it’s possible to use this data with a high degree of confidence, and create prompts when other sources should be used or an onsite inspection initiated.
But for many insurers, the effort involved with managing multiple providers and making sense of what can amount to a mountain of often contradictory data inputs is as maddening as it is impossible on their own. In our view, truly reliable fundamental property data requires a machine learning-based platform capable of fusing traditional data, high-resolution aerial imagery, and a wide range of novel data sources—and turning it into actionable insight on-demand.
Enter: Primary Property Elements
Our next-generation foundational property data combines CAPE’s market-leading geospatial analytics with Foundry, the company’s new data fusion and machine learning platform, to generate AI-verified property data insurers can rely on.
Primary Property Elements are built on technology that ingests and analyzes millions of data records from an expansive array of imagery, traditional property, and unconventional data sources to produce accurate and dependable property insights that can drive better replacement cost estimations and reduce the likelihood of underinsurance.
Its architecture is designed to put a multisource input through an analysis engine that flags inconsistencies and resolves conflicting data. The engine also uses and surfaces confidence scores so carriers can use this data in high-confidence scenarios, while leveraging alternative methods when confidence is low.
Enhanced Customer Experience
The introduction of higher accuracy, AI-powered foundational property data increases the confidence in automatically populating common application fields and reduces the friction a customer may encounter during the process as well as the likelihood of the customer inputting incorrect information. As a result, insurers dramatically streamline the process for agents, as well as underwriters further into the process.
This kind of automation also reduces or eliminates the need for manual reviews and onsite inspections, fueling cost savings while streamlining critical points in the insurance buying or renewal journey. By making the application process faster and more efficient, customers obtain the coverage they need, ultimately leading to increased satisfaction and loyalty.
Improved Accuracy & Consistency
By leveraging CAPE multisource property intelligence to augment or replace existing solutions, underwriters can instantly confirm whether the information in an application is accurate and up-to-date. In turn, this reduces the likelihood of needing to change the replacement cost estimate post-bind.
This includes outlier detection that enables insurers to identify significant errors in the key drivers of replacement cost—including living area, quality grade rating, and the number of stories in the structure. When insurers detect an outlier during the policy quote flow, it can be corrected quickly and easily compared to an endorsement or changed premium on the backend.
These capabilities also help insurers identify existing policies with potentially incorrect Coverage A estimations, enabling them to make necessary corrections across portfolios. The result: faster, more accurate data.
Premium Uplift & Higher Retention Rates
By helping identify over- and under-estimations, CAPE enables insurers to positively impact retention rates. Where existing cost estimations are too low, the insurer has an opportunity to identify them and capture a higher premium while ensuring the customer has the appropriate Coverage A. When they’re too high, the insurer can recalibrate to an appropriately competitive premium.
Because CAPE provides property information from trusted sources, it’s also harder for people to input erroneous information that can lead to a mistakenly higher or lower premium.
Accuracy for a Win-Win
The impact of bringing multisource, AI-driven analysis to these fundamental data points cannot be overstated.
By leveraging advanced machine learning and taking a more granular, multi-dimensional approach to understanding exposure, insurers can price coverage accurately with the speed today’s consumers demand while reducing coverage and pricing mismatches, and tackling the problem of underinsurance.
CAPE’s Primary Property Elements are the first to leverage AI, data science expertise, and a wide range of novel data sources to meet the highest standards of accuracy, enabling carriers to price coverage correctly while verifying that homeowners have the protection they need—instantly.
To learn more about underinsurance, read our post here or visit the Primary Property Elements page here to learn more about our solutions.